In today’s rapidly evolving design landscape, data-driven decision making has emerged as a fundamental pillar of success. This approach allows designers to tap into a wealth of information and empowers them to make informed, strategic choices. The integration of artificial intelligence (AI) has significantly enhanced the ability to analyze and interpret data, transforming the way design strategies are conceived and executed.
The core foundations of data-driven decision making in design
Data-driven decision making involves making informed choices rooted in data analysis rather than relying solely on intuition or personal opinion. In the realm of product design, this approach can draw from various data sources, such as user feedback, market trends, performance metrics, and results from usability testing.
For instance, consider how Airbnb effectively leverages user booking patterns and preferences to enhance their listings. By analyzing this data, they can pinpoint the features that attract specific groups, such as families or solo travelers, focusing on aspects like amenities and sleeping arrangements. This insight allows them to customize their offerings, creating a more personalized experience for users. Additionally, the company employs an AI-powered photo tour that intelligently recognizes and categorizes images by room, helping travelers visualize the house layout more clearly.
Similarly, Nike leverages data through its NikePlus program. By gathering insights from customers’ preferences and activities, Nike can inform product development decisions, such as the creation of the “Nike By You” platform, allowing customers to customize shoes based on their unique tastes.
By tapping into these diverse data sources, designers gain a deeper understanding of user needs and preferences, ultimately leading to products that are not only effective but also resonate more powerfully with their target audiences.
What's the role of AI in data analysis?
AI plays a pivotal role in enhancing data analysis for design strategies by employing machine learning and predictive analytics to process vast datasets with speed and precision. This significantly reduces the time designers spend on manual data interpretation and enables them to focus on crafting innovative solutions.
Moreover, predictive analytics can forecast future behaviors based on historical data trends. When designing a new app feature, a company can use AI to simulate different user scenarios and analyze how changes will likely impact user interactions.
Amazon leverages predictive analytics to enhance its recommendation engine, analyzing users’ past purchase behaviors to suggest products they are likely to buy, ultimately increasing conversion rates. If you’ve ever initiated a general search on Amazon, you may have noticed the “Editorial Recommendations” that appear on the results page. These suggestions, typically positioned below sponsored products and Amazon’s Choice items, are part of the Amazon Onsite Associates Program. This affiliate initiative extends the Amazon Associates program by integrating product-related content from third-party sources directly on Amazon’s platform. This feature facilitates customers in researching and discovering products that align with their interests. By crafting a personalized shopping experience through its recommendation algorithm, Amazon effectively boosts average order values and enhances the revenue generated from each customer.
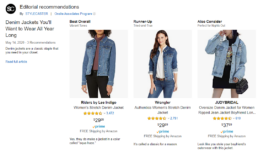
Using AI for data analysis allows designers to pinpoint specific areas of improvement and discover opportunities for innovation. By uncovering hidden insights within complex datasets, AI enables designers to make data-informed decisions that enrich the user experience while driving business success.
Benefits of implementing data-driven strategies
The advantages of adopting data-driven decision making in design are numerous. Firstly, this approach significantly improves the accuracy of design decisions, as choices are grounded in objective data rather than assumptions. Enhanced user satisfaction is another key benefit, as understanding user preferences leads to products that resonate more deeply with target audiences. Additionally, organizations that utilize data-driven strategies often enjoy a competitive edge in the market, as they can rapidly adapt to changing consumer demands and trends.
One notable example is Apple which is known for its focus on user experience and incorporating customer feedback into its product development process. By analyzing customer complaints and feature requests, the company was able to prioritize enhancements that directly addressed user needs, ultimately leading to a significant increase in customer satisfaction and product adoption. The company closely monitors reviews, complaints, and feature requests from users and uses this data to prioritize updates and enhancements across its product line, including iOS updates and app features. This data-driven approach has consistently led to improvements that resonate well with customers, resulting in high levels of satisfaction and strong product adoption rates.
What challenges do designer face when embracing data-driven approaches?
While the benefits are substantial, several challenges can hinder the implementation of data-driven strategies. Issues such as poor data quality, difficulties in data integration from various sources, and a lack of skilled personnel to analyze the data can pose significant barriers. Additionally, resistance to adopting new methodologies within an organization can slow down progress.
In an attempt to personalize marketing efforts, Target developed a predictive model to identify expectant mothers based on purchasing behaviors. While the analysis aimed to provide relevant coupons and recommendations, it inadvertently led to significant backlash.
The data used included items like unscented lotion and prenatal vitamins, which were flagged as indicators of pregnancy. When a teenage girl received targeted ads for baby products, her father discovered the marketing, leading to a confrontation about her potential pregnancy. This incident highlighted not only the ethical implications of leveraging sensitive data but also demonstrated the pitfalls of making assumptions based on data without fully understanding the context or implications of that data. Such experiences underscore the challenge of data quality and interpretation, as well as the risks involved in integrating data-driven methods into design and marketing strategies. Companies must navigate these complexities carefully to ensure that their data-driven approaches are not only effective but also respectful and responsible.
To overcome these challenges, companies can invest in training for their teams to enhance data literacy and cultivate a culture that values data-informed decision-making. Establishing clear protocols for data collection and analysis can also help ensure that the data is both accurate and actionable.
Brands leading the way in data-driven design success
Several organizations have successfully adopted data-driven decision-making strategies in their design processes, yielding remarkable results. For example, Spotify, the music streaming giant employs advanced machine learning algorithms to analyze millions of user interactions on its platform. By delving into factors such as listening habits, song skips, and playlist engagement, Spotify identifies patterns that inform user-centric design choices. A standout example of this is their “Discover Weekly” playlist. Utilizing AI to predict user preferences through complex data analysis, Spotify tailors recommendations to enhance user satisfaction and keep listeners engaged. For more on Spotify’s data-driven approach, check out their “Insights” page.
Coca-Cola also showcases the impact of data analytics in informing their marketing and product development strategies. By examining consumer preferences and purchasing behaviors, Coca-Cola tailors beverage offerings to suit local markets. A prime example is the launch of the “Coca-Cola Freestyle” vending machine, which provides over 100 drink choices, enabling customers to mix and match flavors according to their desires. Learn more about this innovation on Coca-Cola’s official website.
Zalando, a leading online fashion retailer in Europe, utilizes data analytics to improve its supply chain and enhance the customer experience. By employing algorithms to analyze customer preferences and behaviors, Zalando delivers personalized shopping experiences and predictive recommendations. This approach boosts user satisfaction while minimizing return rates.
The integration of advanced technology will enable customers to ask questions and receive relevant suggestions, facilitating a more intuitive navigation of Zalando’s offerings. For example, if a customer inquires, “What should I wear for a wedding in Santorini in July?”, Zalando’s fashion assistant will recognize that it is a formal event and consider the weather conditions in Santorini during that month. Based on this understanding, it will provide product recommendations. In the future, this service may be enhanced by incorporating individual customer preferences, such as favored brands and available sizes, to deliver an even more personalized selection of products.
Unilever applies data analytics for product innovation and to gain consumer insights. Their “Connected 4 Growth” strategy focuses on capturing real-time consumer feedback, enabling quick adaptations to products based on data-driven insights. A notable example is their “Love Beauty and Planet” line, which was launched in response to data highlighting the increasing consumer demand for sustainable and environmentally friendly products. For further information, visit Unilever’s « Sustainable Living » page.
In the ride-sharing sector, Lyft utilizes data to optimize interactions between drivers and passengers. By analyzing usage patterns and customer preferences, Lyft enhances its app features, matches riders with nearby drivers more efficiently, and provides insights to drivers regarding peak demand times. This data-centric strategy has significantly improved the user experience and overall operational efficiency. To learn more about Lyft’s data practices, check their « Safety » page.
Finally, while often thought of as a tech giant, Netflix’s approach to data-driven design is particularly noteworthy. The streaming platform meticulously analyzes viewer behavior, preferences, and engagement metrics to guide content creation and recommendation algorithms. By understanding which shows and movies resonate most with its audience, Netflix can produce original content that is more likely to succeed, demonstrating the impactful synergy between data and creativity. The company also leverage data to create artwork personalization and suggest the best thumbnail that will make you click on the content. For insights into Netflix’s content strategy, explore their Tech Blog.
How can you harness data-driven insights to transform your design process?
Data-driven decision making is reshaping the way designers approach product development and strategy. By leveraging AI to analyze data effectively, organizations can make strategic decisions that enhance user satisfaction, drive innovation, and ensure competitive advantage. As the landscape continues to evolve, embracing a data-informed approach will be essential for designers aiming to create products that resonate profoundly with their audiences. The future of design is not just about creativity; it’s about making impactful decisions grounded in data, enabling designers to craft solutions that truly meet market demands.
This article has been first published in UX Collective
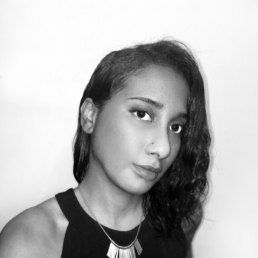
Aurélie Radom
I'm a Lead UX/Product Designer with 10+ years of experience in service design. I love designing meaningful experiences that can change people's lives positively.